Artificial Intelligence (AI) is one of the most transformative technologies of the 21st century. From personal assistants like Siri and Alexa to complex predictive models in healthcare and finance, AI is reshaping how we live and work. However, with great power comes great responsibility. As AI becomes more deeply integrated into critical systems, the need for robust cybersecurity measures has never been more urgent. Cybersecurity in AI is about safeguarding data, algorithms, and the very integrity of AI systems from potential threats and vulnerabilities.
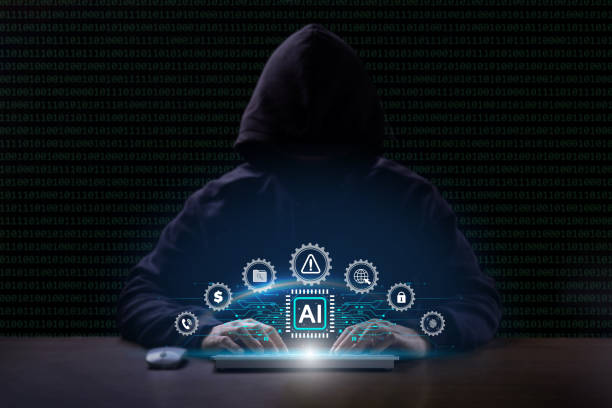
The Importance of Cybersecurity in AI
- Data Protection: AI systems rely heavily on data to function effectively. This data can include personal information, financial records, and even classified information. A breach in an AI system could expose sensitive data to malicious actors, leading to significant personal and organizational risks. For instance, if an AI-powered financial system were hacked, it could lead to financial fraud or the theft of millions of dollars.
- System Integrity: AI systems make decisions based on the data and algorithms they are trained on. If these are compromised, the outcomes can be catastrophic. For example, an autonomous vehicle relying on tampered AI could misinterpret road conditions, leading to accidents. Securing AI ensures that its decisions remain accurate and trustworthy.
- Preventing Manipulation: Cybercriminals can manipulate AI models through adversarial attacks, injecting false data to mislead the system. For example, a facial recognition system could be tricked into misidentifying individuals, leading to security breaches.
- Ethical Concerns: Protecting AI systems from misuse ensures that they are used ethically. AI-driven cybersecurity failures can lead to discrimination, misinformation, and other ethical dilemmas.
Common Cyber Threats to AI Systems
- Adversarial Attacks: These involve feeding AI systems misleading or malicious inputs to produce errors. For example, attackers could use manipulated images to fool AI-powered security cameras.
- Data Poisoning: This occurs when attackers inject false or corrupted data into an AI system during its training phase. This can compromise the system’s ability to function correctly.
- Model Inversion Attacks: In this type of attack, cybercriminals attempt to extract sensitive information from AI models, such as the training data used to develop them.
- Unauthorized Access: Weak cybersecurity measures can allow unauthorized individuals to gain access to AI systems, enabling them to alter algorithms, steal data, or disrupt operations.
- Supply Chain Attacks: AI systems often depend on third-party software, hardware, or data sources. Compromising any part of this supply chain can introduce vulnerabilities into the AI system.
Key Strategies to Enhance Cybersecurity in AI
- Implement Robust Data Security Measures: Protecting the data that AI systems use is crucial. This includes encrypting sensitive data, using secure data storage solutions, and ensuring data integrity through regular checks.
- Secure AI Models: AI models themselves should be treated as sensitive assets. Techniques like differential privacy and federated learning can help protect AI models from being reverse-engineered or tampered with.
- Regular Updates and Patching: Keeping AI software and systems updated ensures that they are protected against known vulnerabilities. Developers should also provide regular patches to fix emerging security issues.
- AI-Driven Security Tools: Organizations can use AI itself to enhance cybersecurity. AI-powered tools can monitor systems for unusual activity, detect potential threats in real time, and respond to incidents more effectively.
- Adversarial Testing: Testing AI systems against adversarial attacks can help identify vulnerabilities. This allows organizations to strengthen their defenses before real-world threats emerge.
- Educate and Train Teams: Human error is often a significant factor in cybersecurity breaches. Ensuring that teams are trained in best practices for securing AI systems can minimize risks.
Real-World Applications of Cybersecurity in AI
- Healthcare: AI systems in healthcare, such as diagnostic tools and patient management systems, deal with highly sensitive data. Cybersecurity ensures that patient information remains confidential and that AI-powered diagnostics are not manipulated.
- Finance: AI is widely used in finance for fraud detection, algorithmic trading, and risk assessment. Securing these systems is critical to prevent financial losses and maintain trust.
- Transportation: Autonomous vehicles rely on AI for navigation and decision-making. Cybersecurity measures ensure these systems are not compromised, preventing accidents and ensuring passenger safety.
- Defense: Military applications of AI, such as autonomous drones and threat detection systems, require the highest levels of cybersecurity to prevent adversaries from exploiting vulnerabilities.
Future Challenges and Opportunities
- Evolving Threats: As AI becomes more advanced, so do the methods used by cybercriminals. Staying ahead of these threats requires constant innovation in cybersecurity technologies.
- Regulatory Compliance: Governments and regulatory bodies are introducing new standards for AI and cybersecurity. Organizations must adapt to these evolving requirements to remain compliant.
- Ethical AI Development: Incorporating ethical considerations into AI design and security measures can help prevent misuse and build public trust in AI technologies.
- Collaboration Between Sectors: Governments, private organizations, and academic institutions must work together to develop and implement effective cybersecurity strategies for AI.
Conclusion
Cybersecurity in AI is not just an option; it’s a necessity. As AI continues to integrate into every aspect of our lives, protecting these systems from cyber threats is crucial for maintaining their integrity, reliability, and trustworthiness. By implementing robust security measures and staying vigilant against evolving threats, we can ensure that AI remains a force for good, driving innovation while keeping data and systems safe.